

This eventually helps receive game-changing and revolutionary business insights. Using properly cleaned and formatted data while building data models or applications will ensure top-quality reporting and analysis with proper accuracy. These errors will be much more difficult to catch and fix after the data has been transferred out of its original format. Preparing data for analysis will help avoid mistakes, saving more time to be invested down the line.
KLIB LIBRARY PYTHON FREE
It must be clean and free of errors before using data for analysis or plugging it into dashboards for visualizations. A thorough data preparation process can give an organization many advantages and rather give it a headstart. This data can be stored or inculcated into a third-party application, such as a business intelligence tool, clearing the way for processing and analysis. Once all the mentioned processes are done, the data is prepared. Then, data is further transformed by updating the format or value entries to reach a clean and well-defined outcome or to make the data more easily understood by a wider spectrum of audiences. That’s why it’s important to perform data cleansing and taking care of the data regularly. The data cleansing process is done all at once and can take quite a while if the information has been piling and stacking up for years. The Data cleansing process usually also involves cleaning up all the data compiled previously in one area.
KLIB LIBRARY PYTHON UPDATE
Data cleansing is a process where you go through all of the data to be processed and either remove or update information that is considered to be incomplete, incorrect, improperly formatted, duplicated, or highly irrelevant.
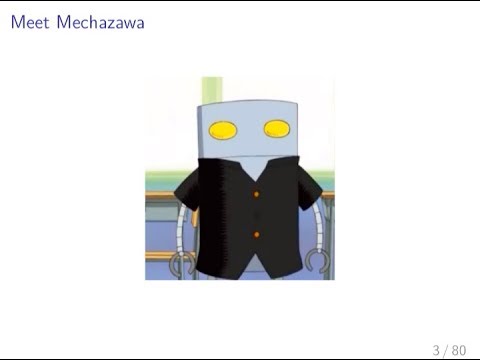
Cleaning up the data is traditionally another most time-consuming part of the data preparation process, but it’s crucial for removing forward and dealing with faulty data to help fill in the gaps present. This step is essential and helps get to know the data and understand what has to be done before the data can be called useful in a particular context. After the data is collected, it is important to discover and explore each dataset to prepare and process.

This can come from an existing data catalogue, warehouse or can be added ad-hoc. The data preparation process first begins with finding the right data. Data preparation is often considered a lengthy undertaking for data professionals or business users, but it is an essential prerequisite to put data in context to turn it into insights that might help in decision making, eliminating the bias resulting from poor data quality. It is a small yet important step before processing and often involves reformatting the data, making corrections, and combining multiple data sets to enrich the present data. Data preparation is the process of cleaning and transforming the raw data before preprocessing and analysis. Lately, the process of Data Preparation has gotten easier with new tools and technologies that enable anyone to cleanse and clarify data independently. A good data preparation procedure allows for efficient analysis, limits and minimizes errors and inaccuracies that can occur during processing, making the processed data more accessible to all users. With such underlying concerns, the method of Data Preparation becomes very helpful and a crucial aspect to begin with. Collecting and managing data properly and the methods used to do so play an important role. Big Data comes along with its complications.
